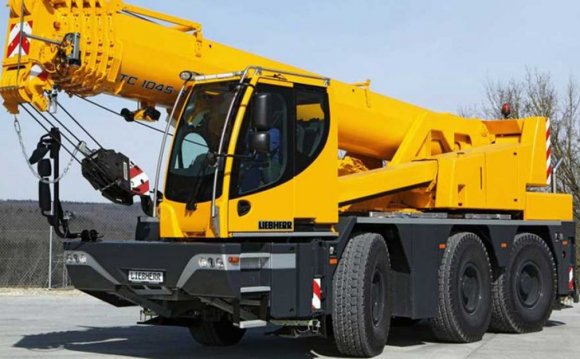
Building devices that uncover and think like men and women Lake et al., arXiv 2016
Pro-tip: if you’re planning to attempt to read and write-up a paper every weekday, it's most readily useful not to ever choose papers that run to over 50 pages. As soon as the report is really as interesting as “Building machines that learn and believe like individuals” however, it's a pleasure to take some extra time. You could recall from Monday’s write-up of “AI and lifestyle in 2030” that alongside constant development, we harbour a secret hope that we get one or higher big advancements on the after that decade. In which might those advancements result from? I’m certain we’ll manage to accept dilemmas of increasing scale, but We suspect progress there may feel much more progressive. The locations where we're able to however make order-of-magnitude design improvements appear to be:
- Data performance – training a design to a particular level of skills making use of less instruction data than today’s designs require.
- Training time – closely correlated to data effectiveness, achieving a particular level of proficiency with considerably decreased instruction time.
- Adaptability – to be able to better make use of prior ‘knowledge’ (skilled models) whenever mastering a unique task (which in addition indicates requiring less information, and faster instruction times of training course). (See e.g. “Progressive neural communities” ).
Plus I hope, several wonderful surprises coming out of study groups and professional labs. ‘Building machines that uncover and think like folks’ investigates several of those questions by asking how humans appear to discover, in which we nevertheless outperform advanced device mastering methods, and why that might be. It’s in an equivalent vein to “Towards deep symbolic reinforcement learning“, one of my favourite reports from last couple of weeks.
For those of you short promptly, right here’s the TL;DR variation through the abstract:
We review development in cognitive technology recommending that certainly human-like learning and thinking machines will have to attain beyond current manufacturing styles both in what they learn, and exactly how they understand it. Especially, we argue that these devices should (a) develop causal different types of society that help description and understanding, as opposed to merely solving structure recognition issues; (b) floor mastering in intuitive theories of physics and psychology, to aid and enrich the knowledge that's learned; and (c) harness compositionality and learning-to-learn to rapidly get and generalize understanding to brand-new tasks and situations.
You’ll be missing a lot if you just stop there though.
Pattern recognition vs model creating
Like Garnelo et al., Lake et al. see an important distinction between discovering methods being fundamentally predicated on statistical pattern recognition, and learning methods that develop some type of the entire world they can reason over.
The design recognition method discovers functions having some thing in common – category labels for example – across a big diverse collection of education data. The design building method creates designs to comprehend and explain the world, to imagine effects of actions, and make plans.
The essential difference between pattern recognition and model-building, between prediction and explanation, is main to your view of peoples cleverness. Just like boffins look for to spell out nature, not simply predict it, we come across human being idea as basically a model-building activity.
Two challenges that reveal present restrictions
In intellectual technology, your head is certainly not perceived as getting started as a collection of general-purpose neural networks with few preliminary constraints. Instead, (many) intellectual scientists think we start off with a number of early inductive biases offering primary principles particularly number, area, company, and items, plus discovering formulas that rely on previous understanding to draw out understanding from small amounts of instruction data. Lake et al. provide two simple challenge conditions that emphasize a few of these distinctions.